Digital Dilemma: Majority of Executives Stumble in AI Preparedness Race
Business
2025-04-23 12:00:48Content
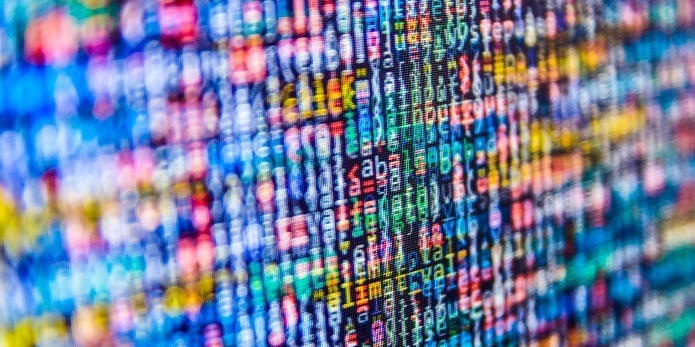
A groundbreaking global audit has revealed a stark reality: major corporations worldwide are struggling to harness the transformative potential of Generative AI due to significant data readiness challenges. The comprehensive study exposes a critical gap between companies' AI ambitions and their actual data infrastructure preparedness.
Despite the immense promise of Generative AI to revolutionize business operations, organizations are grappling with fundamental data quality and management issues. The audit uncovered that many enterprises lack the robust, clean, and structured data necessary to effectively deploy advanced AI technologies.
Key findings highlight a widespread lack of confidence among corporate leaders in their existing data ecosystems. Companies are recognizing that successful AI implementation requires more than just cutting-edge technology—it demands a strategic approach to data governance, quality control, and organizational alignment.
This revelation underscores the urgent need for businesses to invest in comprehensive data transformation strategies. Organizations must prioritize data cleansing, integration, and standardization to unlock the full potential of Generative AI and maintain competitive advantage in an increasingly AI-driven marketplace.
Navigating the AI Revolution: Corporate Data Preparedness in the GenAI Era
In the rapidly evolving landscape of technological innovation, businesses worldwide are grappling with a critical challenge that could define their competitive edge: preparing their data infrastructure for the transformative potential of Generative Artificial Intelligence. As organizations seek to harness the unprecedented capabilities of GenAI, a global audit reveals a complex and nuanced picture of corporate readiness that demands immediate strategic attention.Unlocking Potential: The Make-or-Break Moment for Enterprise AI Transformation
The Data Quality Conundrum
Enterprises are confronting a fundamental paradox in their artificial intelligence journey. Despite massive investments in digital transformation, many organizations find themselves wrestling with fundamental data quality challenges that threaten to derail their GenAI ambitions. The audit uncovers a startling reality: confidence in data infrastructure is alarmingly low, with numerous corporations struggling to establish the robust, clean, and structured data ecosystems required for meaningful AI integration. The complexity of this challenge extends far beyond simple technical limitations. It represents a profound strategic inflection point where traditional data management approaches collide with the exponential demands of generative technologies. Companies must now reimagine their entire approach to data collection, curation, and governance, recognizing that the quality of their AI outputs is intrinsically linked to the integrity of their input data.Strategic Implications of Data Readiness
The global audit reveals that data readiness is not merely a technical consideration but a critical business imperative. Organizations that successfully navigate this transition stand to gain unprecedented competitive advantages, while those lagging behind risk becoming obsolete in an increasingly AI-driven marketplace. The most forward-thinking enterprises are developing comprehensive data strategies that go beyond traditional IT frameworks, integrating cross-functional expertise and creating adaptive, intelligent data ecosystems. This strategic transformation requires a holistic approach that encompasses technological infrastructure, organizational culture, and human capital. Companies must invest in not just technological solutions, but in building a data-literate workforce capable of understanding, managing, and leveraging advanced AI technologies effectively.Technological and Cultural Barriers to GenAI Adoption
The path to GenAI readiness is fraught with complex technological and cultural challenges. Many organizations discover that their existing technological architectures are fundamentally misaligned with the requirements of generative AI systems. Legacy systems, fragmented data repositories, and siloed organizational structures create significant barriers to effective AI implementation. Cultural resistance presents an equally formidable obstacle. Many traditional enterprises struggle to cultivate the innovative, experimental mindset required to truly embrace generative AI technologies. This requires a fundamental reimagining of organizational learning, risk tolerance, and technological adaptation.Emerging Best Practices in Data Preparation
Leading organizations are developing sophisticated approaches to data preparation that transcend traditional methodologies. These strategies involve comprehensive data auditing, advanced cleaning techniques, and the development of dynamic, self-evolving data infrastructures that can support the complex demands of generative AI systems. Key emerging practices include implementing robust data governance frameworks, investing in advanced machine learning data preparation tools, and creating cross-functional teams dedicated to data quality and AI readiness. The most successful enterprises are treating data not as a static resource, but as a dynamic, strategic asset that requires continuous refinement and strategic investment.The Global Competitive Landscape
The global audit highlights significant variations in GenAI readiness across different regions and industry sectors. Technology-forward markets like Silicon Valley and emerging innovation hubs in Asia are demonstrating more aggressive and sophisticated approaches to data preparation and AI integration. However, the most successful organizations are not defined by their geographic location, but by their ability to create adaptive, learning-oriented data ecosystems that can rapidly evolve in response to technological change. This requires a combination of technological investment, strategic vision, and a culture of continuous innovation.RELATED NEWS
Business
Trade Tensions Escalate: Trump Hints at Potential Tariff Hikes on USMCA Partners
2025-03-09 16:16:54
Business
Travel Giant American Express GBT Reshapes Industry with Bold CWT Merger Move
2025-03-21 20:48:00
Business
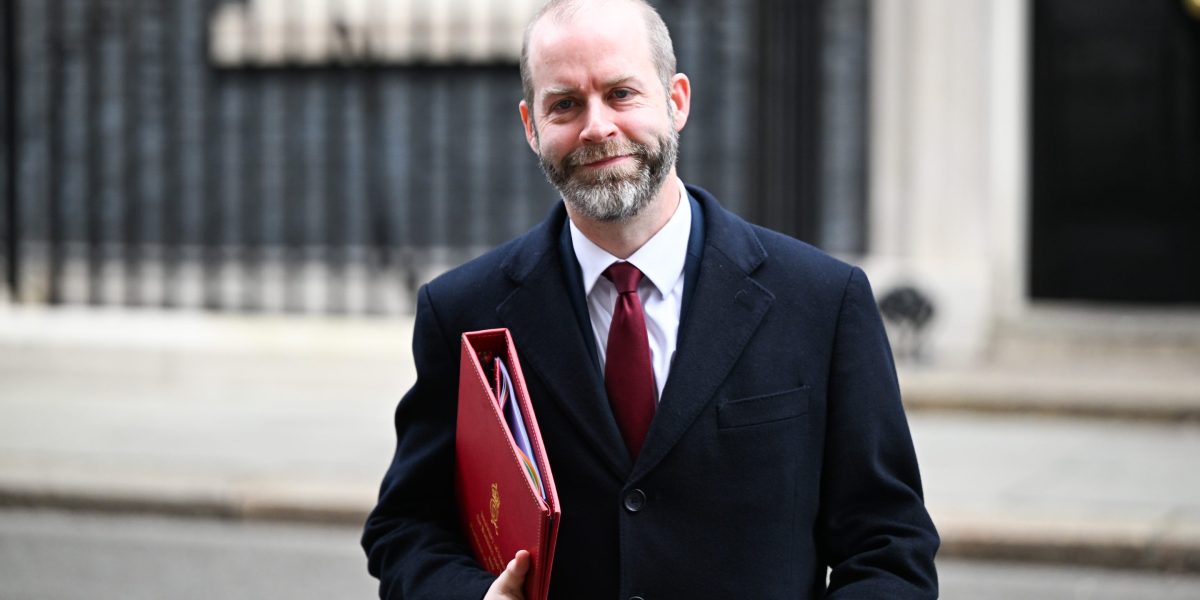
Steel Showdown: British Minister's High-Stakes DC Mission to Shield UK Manufacturers from Trump's Tariff Threat
2025-03-18 10:42:33